自然语言处理领域近几年飞速发展,在翻译、电商、金融等众多领域都实现了跨越式的进步并催生了很多改变日常生活的应用。
深圳市计算机学会特邀三位入选2021年ACL的论文作者分享入选论文,交流NLP领域研究方法、应用发展方向,探讨检索式对话系统中的回复选择、机器翻译等研究内容。
会议流程如下:
一、主办单位
深圳市计算机学会
二、活动时间
7月初(具体时间安排视疫情情况确定)
三、活动地点
深圳大学城
四、活动安排
活动环节 | 讲者 | 时间 |
签到入场 | 14:00-14:30 | |
宣讲主题介绍 | 14:30-14:40 | |
论文题目:《Dialogue Response Selection with Hierarchical Curriculum Learning》;《BoB: BERT Over BERT for Training Persona-based Dialogue Models from Limited Personalized Data》 | 王琰,腾讯AI Lab高级研究员;ACL、EMNLP、NAACL、AAAI等顶级会议发表论文二十余篇;主导开发了腾讯AI开放平台智能闲聊服务。 | 14:40-15:20 |
论文题目:《Fast and Accurate Neural Machine Translation with Translation Memory》;《Neural Machine Translation with Monolingual Translation Memory》 | 刘乐茂,腾讯AI Lab高级研究员;ACL、EMNLP、NAACL、AAAI等顶级会议发表论文约四十余篇;曾担任IJCAI2021 高级程序委员和Findings of EMNLP2020 论文出版联合主席。 | 15:20-16:00 |
论文题目:《GWLAN: General Word-Level AutocompletioN for Computer-Aided Translation》;《Data Augmentation for Text Generation Without Any Augmented Data》 | 李华阳,腾讯AI Lab研究员;在ACL、EMNLP、AAAI等自然语言处理相关会议和刊物上发表论文十余篇。 | 16:00-16:40 |
圆桌交流 | 16:40-17:00 |
五、嘉宾简介及论文摘要
王琰,博士,毕业于香港城市大学,现担任腾讯公司人工智能实验室(AI Lab)高级研究员,主要负责智能闲聊和文本生成相关的研究与算法工作。主导开发了腾讯AI开放平台智能闲聊服务,为腾讯数十款智能音箱、客服以及智能NPC产品提供千人千面的闲聊能力,并在ACL、EMNLP、NAACL、AAAI等自然语言处理和机器学习顶级会议发表论文二十余篇。
1.宣讲论文题目:《Dialogue Response Selection with Hierarchical Curriculum Learning》
2.宣讲论文摘要:We study the learning of a matching model for dialogue response selection. Motivated by the recent finding that models trained with random negative samples are not ideal in real-world scenarios, we propose a hierarchical curriculum learning framework that trains the matching model in an “easy-to-difficult” scheme. Our learning framework consists of two complementary curricula: (1) corpus-level curriculum (CC); and (2) instance-level curriculum (IC). In CC, the model gradually increases its ability in finding the matching clues between the dialogue context and a response candidate. As for IC, it progressively strengthens the model’s ability in identifying the mismatching information between the dialogue context and a response candidate. Empirical studies on three benchmark datasets with three state-of-the-art matching models demonstrate that the proposed learning framework significantly improves the model performance across various evaluation metrics.
3.中文概要:本文由腾讯主导,与剑桥大学和香港中文大学合作完成。本文主要研究领域为检索式对话系统中的回复选择问题。由于最近同行们研究发现使用随机负样本训练的模型在现实世界中的场景中并不理想,我们提出了一种层次课程学习框架,以“从易到难”的顺序训练回复选择模型。该框架包括两种不同课程:语料级课程和实例级课程。在语料级课程中,该模型逐渐学习到了如何找到与对话上文匹配的回复,而在实例级课程中,模型逐渐学习到了如何发现与对话上文不匹配的回复。我们用层次课程学习框架训练了三个最新的回复选择模型,并在三个标准数据集上验证其效果,实验表明,本文提出的学习框架使模型在各种评价指标上都获得了显著的提升。
刘乐茂,现任腾讯公司人工智能实验室(AI Lab)高级研究员,主要从事自然语言处理和机器翻译相关的研究与开发工作;2013年博士毕业于哈尔滨工业大学,随后在纽约城市大学和日本国立信息通讯研究机构(NICT)从事机器翻译方面的研究工作;在ACL、EMNLP、NAACL、ICLR、AAAI等自然语言处理相关会议和刊物上发表论文约四十篇,曾担任IJCAI2021 高级程序委员和Findings of EMNLP2020 论文出版联合主席。
1.宣讲论文题目:《Fast and Accurate Neural Machine Translation with Translation Memory》
2.论文摘要:It is generally believed that a translation memory (TM) should be beneficial for machine translation tasks. Unfortunately, existing wisdom demonstrates the superiority of TM-based neural machine translation (NMT) only on the TM-specialized translation tasks rather than general tasks, with a non-negligible computational overhead. In this paper, we propose a fast and accurate approach to TM-based NMT within the Transformer framework: the model architecture is simple and employs a single bilingual sentence as its TM, leading to efficient training and inference; and its parameters are effectively optimized through a novel training criterion. Extensive experiments on six TM-specialized tasks show that the proposed approach substantially surpasses several strong baselines that use multiple TMs, in terms of BLEU and running time. In particular, the proposed approach also advances the strong baselines on two general tasks (WMT news Zh -> En and -> De)
3.中文概要:翻译记忆能帮助提升机器翻译质量。遗憾的是,现有基于翻译记忆的翻译模型在通用翻译任务上无效,它只在针对翻译记忆研究的特定任务上有效,并且具有不可忽略的计算开销。因此,本文提出了一种高效且准确的融合翻译记忆的神经翻译模型。此模型的架构简单,它仅使用一个双语句子作为其翻译记忆,因而它的训练和推理相当高效;更重要的是,我们还提出了一种新的训练方法来优化模型参数。我们在6个针对翻译记忆研究的特定任务上进行实验,结果表明:相比于几种使用多个双语句子作为翻译记忆的强基线系统,该模型在翻译质量和运行时间方面有极大的优势;特别地,该模型在两个通用任务(WMT Zh->En和En ->De)上也能提升翻译质量。
李华阳,现任腾讯公司人工智能实验室(AI Lab)研究员,主要从事自然语言处理以及(交互式)机器翻译相关的研究与落地工作;主导研发了腾讯交互式机器翻译系统 TranSmart,目前已为联合国在内的十余家大型机构及公司提供辅助翻译服务;在ACL、EMNLP、AAAI等自然语言处理相关会议和刊物上发表论文十余篇。
1.部分宣讲论文题目:《GWLAN: General Word-Level AutocompletioN for Computer-Aided Translation》
2.论文摘要:Computer-aided translation (CAT), the use of software to assist a human translator in the translation process, has been proven to be useful in enhancing the productivity of human translators. Autocompletion, which suggests translation results according to the text pieces provided by human translators, is a core function of CAT. There are two limitations in previous research in this line. First, most research works on this topic focus on sentence-level autocompletion (i.e., generating the whole translation as a sentence based on human input), but word-level autocompletion is under-explored so far. Second, almost no public benchmarks are available for the autocompletion task of CAT. This might be among the reasons why research progress in CAT is much slower compared to automatic MT. In this paper, we propose the task of general word-level autocompletion (GWLAN) from a real-world CAT scenario, and construct the first public benchmark to facilitate research in this topic. In addition, we propose an effective method for GWLAN and compare it with several strong baselines. Experiments demonstrate that our proposed method can give significantly more accurate predictions than the baseline methods on our benchmark datasets.
3.中文概要:计算机辅助翻译(CAT),即在翻译过程中使用软件来协助人工翻译,已被证明有助于提高人工译员的生产力。其中根据人工译员提供的文本片段提示翻译结果的自动补全功能是CAT的核心。此前在这方面的研究有两个限制。首先,关于这个方向的大多数研究工作都集中在句子级的自动补全(即根据人工译员的输入生成整个译文),但到目前为止,词级自动补全还没有被充分探索。其次,几乎没有公开的基准可用于CAT的自动补全任务。这可能是CAT的研究进展比自动翻译慢的原因之一。在本文中,我们从真实的CAT场景中提出了一个通用词级自动补全任务(GWLAN),并构建了第一个公开基准以促进该领域的研究。此外,我们为GWLAN任务提出了一种简单有效的方法,并将其与几个基线进行比较。实验证明,在构建的基准数据集上,我们提出的方法可以比基线方法提供更准确的预测。
六、报名参会
扫码添加微信进入宣讲会讨论群(备注学校+姓名):
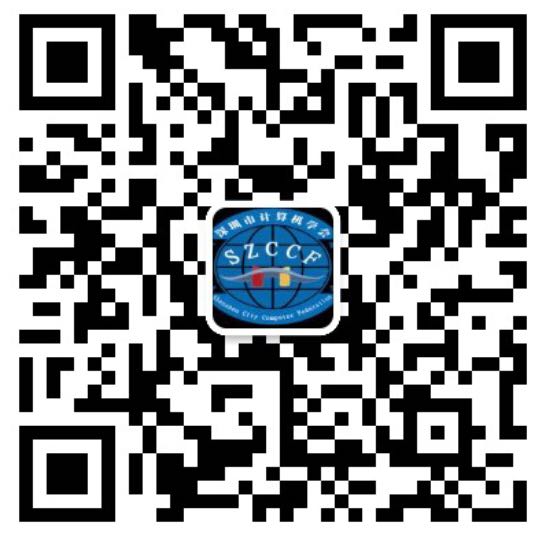
联系微信:ICNLAB
联系电话:王宏刚 17898468114